EECS 349 Machine Learning - Course Project
Attributes Recognition of Apparel
Project members
Xiaoyi Liu, Wenyu Han, Qingliang Zhao
Contact email address
xiaoyiliu2021@u.northwestern.edu
wenyuhan2017@u.northwestern.edu
qingliangzhao2019@u.northwestern.edu
Course
EECS 349 Machine Learning, Northwestern University
Course Website:http://www.cs.northwestern.edu/~ddowney/courses/349_Fall2018/
Instructor: Doug Downey
Abstract
Our task is to recognize the hierarchical attributes tree of the piece of cloth based on the photo of it. The algorithm worked as a structured classifier, to describe the cognitive process of apparel. Apparel knowledge system can be applied to a broad range of application scenarios, such as apparel image retrieval, label navigation, apparel match, etc.
We used Convolutional Neural Network as our basic learning method, specifically, utilizing InceptionV3 model (performs better than VGG16) on Keras.
In order to get our result in acceptable time, we used shared weight to train the 8 category in a single model. We got a validation accuracy of 74.94% eventually. To achieve higher accuracy, we plan to try data augmentation methods. we used random-cropping, rotating and Gauss noise as our data augmentation methods.
Outline of Detailed Report
1. Task description: What and Why
2. Dataset: Source and details
3. Learning process: method and key result
4. Conclusion and suggestions for future work
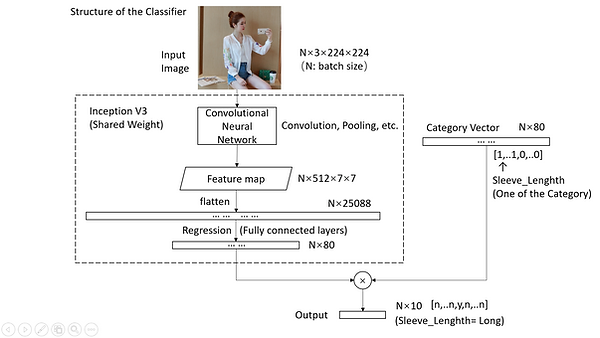
Task description: What and Why
According to official statistics from different countries, the market value of the global apparel market is worth over USD 3 trillion. Although artificial intelligence (AI) technology has been evolving along with the fashion industry, there are still different challenges in different areas that need to be addressed. One of those major challenges is apparel attributes recognition. Apparel attributes are the basic knowledge of fashion field, which are large and complex.
Our task is to recognize the hierarchical attributes tree of the piece of cloth based on the photo of it. The algorithm worked as a structured classifier, to describe the cognitive process of apparel. Apparel knowledge system can be applied to a broad range of application scenarios, such as apparel image retrieval, label navigation, apparel match, etc.
For example, A potential application of our attribute recognition algorithm is a “Smart Outfit Assistant”. About 60% of responders have more than 50 pieces of cloth and more than 50% of the responders take more than 5 minutes to decide what to wear everyday according to our customer survey. We can develop an application based on the structured data of the user’s cloth and give suggestion on his daily outfits based on the occasion, weather etc. to help them making decision.
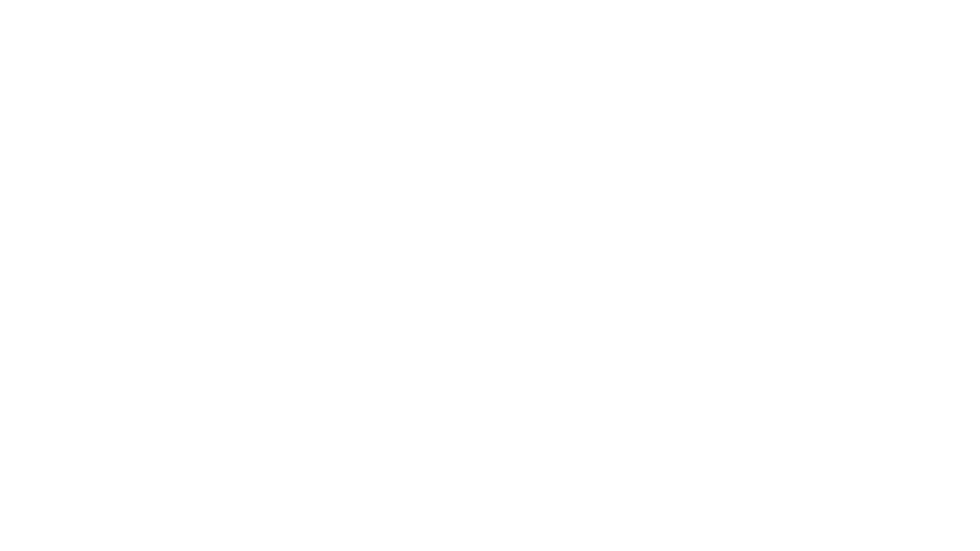
Dataset: Source and details
The FashionAI Global Challenge provided a systematic and practical apparel attributes recognition dataset. The hierarchical clothing label system contains a professional arrangement of the fundamental apparel attributes. Currently, it covers 5 women clothing categories (41 sub-categories in total), with 8 dimensions of 54 attributes labels. There are totally 257,000 annotated images in this dataset.
All image data are from Alibaba e-Commerce platform, and are labeled by well-trained annotators. These labels are then double-checked by fashion experts to guarantee a high labeling accuracy. We partitioned the data randomly for the purpose of training/validation/testing as 180, 000/7,000/70, 000 annotated images respectively.
The labels in training dataset contains Attribute Dimension(AttrKey) and Attribute Value (AttrValues).
a) AttrKey: A specific apparel attribute, e.g. sleeve length. There are nine values in the sleeves length dimension: invisible, sleeveless, cup sleeve, short sleeve, mid length, 3/4 sleeve, wrist length sleeve, long sleeve and extra-long sleeve.
b)AttrValues: The specific value under a specific attribute dimension, e.g. short-length and mid-length under the dimension of sleeve length. the “nnnnnnmyn” annotation in the figure contain nine digits in total, with each digit representing one of the three letters : y(means “yes”, “must be”), m(means “may be”, “probably”), and n(means “no”, “must not be”)


Figure 1. Demonstration of the apparel attributes of training data[1]
Learning process: method and key result
-
Learning method
As we are focusing on large-scale image recognition, we chose Convolutional Neural Network as our basic learning method, specifically, utilizing different models on Keras
Our initial approach is to train a Convolutional Neural Network focused on the first attribute dimension, sleeve_length_lables. We utilized VGG16 model[2] and trained about 8000 images. It took us 20 hours to finish the training and achieved a accuracy of 87.7% on validation dataset. The accuracy was satisfiable yet the training time is not affordable for us to get all 8 categories trained.
-
Model selection
To solve the problem, our next step was to try shared weight method to train the 8 category in a single model so that we want much less training time and smaller model size in total. The next step was to try different models on Keras and find a better one.
Documentation for VGG16 and InceptionV3 models[3]
For VGG 16, it took 61 minutes to get through a single epoch. The model size is approximately 1.0 G and achieved 74% validation accuracy after about 10 epoches. While InceptionV3 only needs 50 minutes to get through a single epoch and the model size is only 180 M and achieved 75% validation accuracy. Overall, InceptionV3 has a better performance compared with VGG 16.
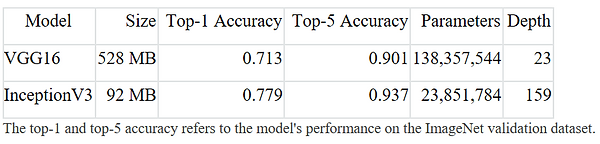
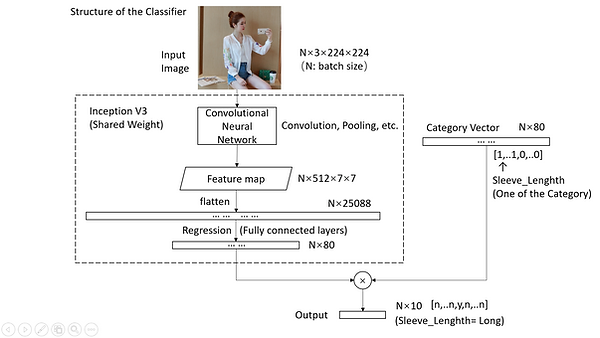
Figure 3. Learning curve of the Inception V3 classifier
-
Data augmentation
To achieve higher accuracy, we tried data augmentation methods to enlarged our data set. we used random-cropping, rotating and Gauss noise as our data augmentation methods. Since the data set was enlarged to 20 times, it took 80 hours to finish the training. Although we finished the code of data augmentation, since it takes too much time and we don't have enough calculating resource, the training is not finished yet, but it supposed to get a higher validation accuracy.